The Problem with Current Speech Recognition Technology
To gain trust and continue accelerated consumer and business adoption of voice technology, ASR system engineers—and voice-enabled device makers—must provide the absolute best ASR performance possible.
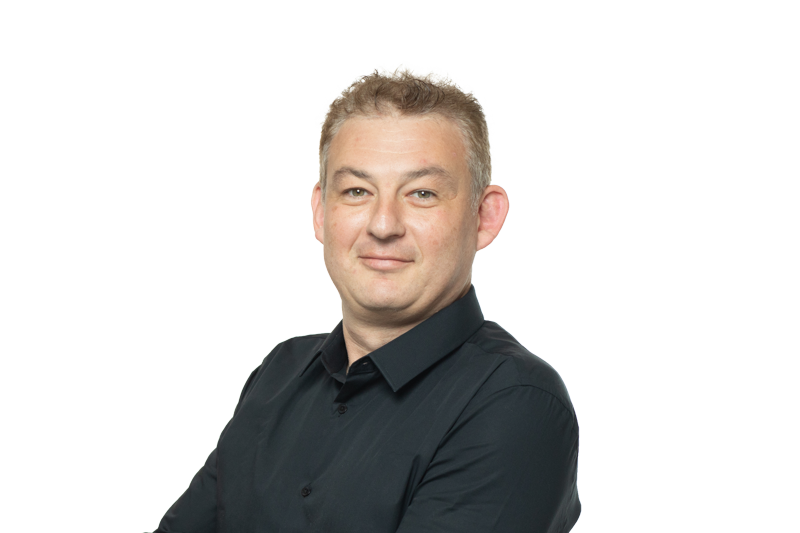
Automatic speech recognition (ASR) engines have been around for more than three decades. The technology rapidly went from clunky, costly, slow dictation applications to artificial intelligence-driven speech recognition devices in our cars, homes, classrooms, and work.
The explosion in speech recognition adoption by consumers and businesses occurred when Apple introduced the Siri-enabled iPhone 4S in 2011. At that time, the global voice and speech technology market was estimated at $600 million. The market reached $8.3 billion in 2021, and forecasters expect it to reach an astounding $22.2 billion by 2027.
Despite the predictions of triple growth in the voice and speech technology industry, the problems of poor speech recognition performance—systems that cannot perform in noisy environments, amid interfering signals, and that cannot accurately identify who is talking — may slow this exponential growth.
Consumer Frustration with ASR Technology
In a 2020 worldwide survey, 73 percent of users say accuracy was the number one factor inhibiting voice tech adoption.
Accent and dialect-related issues are the second most frustrating issues users face. End-user expectations and complexity of use and integration are also leading barriers to voice tech adoption.
The following quote from a PwC study exemplifies the current frustration with speech recognition devices and a significant obstacle: Trust.
“The assistant can’t answer my questions half the time, but I’m supposed to trust it to help me with something involving money?”
—Female, 26, PwC
A recent study by Voicebot.ai shows a sharp decline in smart speaker use in the past two years. Instead, consumers are using their smartphone virtual assistants more.
Can this be partly attributed to customer frustration with voice recognition capabilities in smart speakers? That a smartphone will understand a user more easily might be attributed to users holding a phone closer or using earbuds that bring the users’ voices closer to the speech recognition system.
Poor speech recognition performance frustrates consumers. ASR systems are not accurately processing and understanding human speech due to background noise, multiple people talking, signal disruption, and distance.
The ideal ASR system offers accurate speech recognition in quiet or chaotic environments. Additionally, the consummate voice recognition device will know who is speaking and where they are located to provide accurate and personalized responses to voice commands.
To gain trust and continue accelerated consumer and business adoption of voice technology, ASR system engineers—and voice-enabled device makers—must provide the absolute best ASR performance possible.
Addressing ASR Technical Challenges
Voice-enabled devices have the potential to revolutionize many aspects of our lives, from home automation to assistive and cognitive assistance.
Many businesses deploy voice interfaces to improve the customer experience and boost brand engagement. Voice interfaces may also increasingly be used for customer service and support or for other purposes such as streamlining the health and financial sectors as voice recognition and speech synthesis becomes more accurate and easier to use.
In the IT industry, voice is not new. But the increasing popularity and availability of voice-enabled smartphones, coupled with the growing demand for more natural-sounding human-machine interactions, has made it a top priority for many software firms.
The technical challenges associated with voice recognition have been well known and tackled by many companies over the years. The market for voice-enabled devices will continue to grow as long as we address these challenges.
Study Shows Kardome Delivers 95% Speech Recognition Accuracy in Challenging Soundscapes
The accompanying study shows that Kardome’s voice user interface technology outperforms traditional speech recognition algorithms in the noisiest soundscapes.
The study measures Wake Word False Rejection Rates (FRR) and Response Accuracy Rates (RAR) by studying ASR performance in various environments, from the quietest to the noisiest.
We tested FRR and RAR using a smart speaker placed in a typical noisy living room environment with background noises that include fans, air conditioning, or children playing. In addition, we conducted testing with the smart speaker placed next to a loud, smart TV.
Download the study
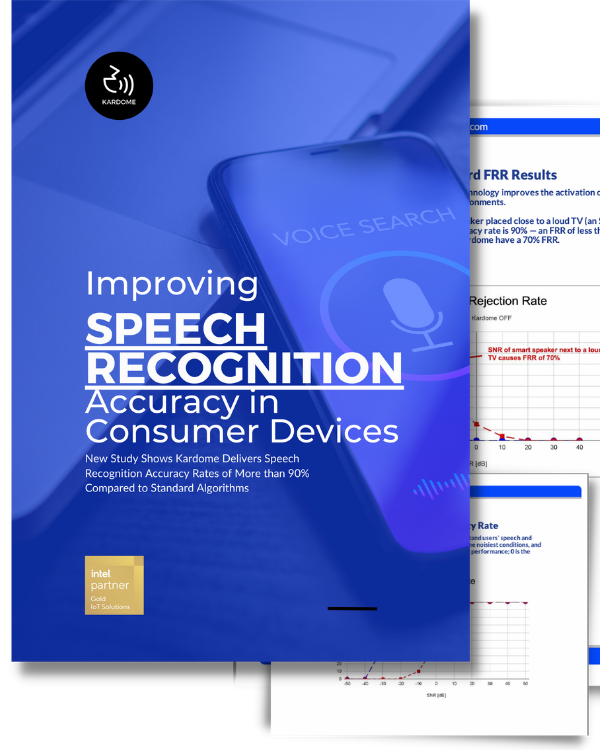